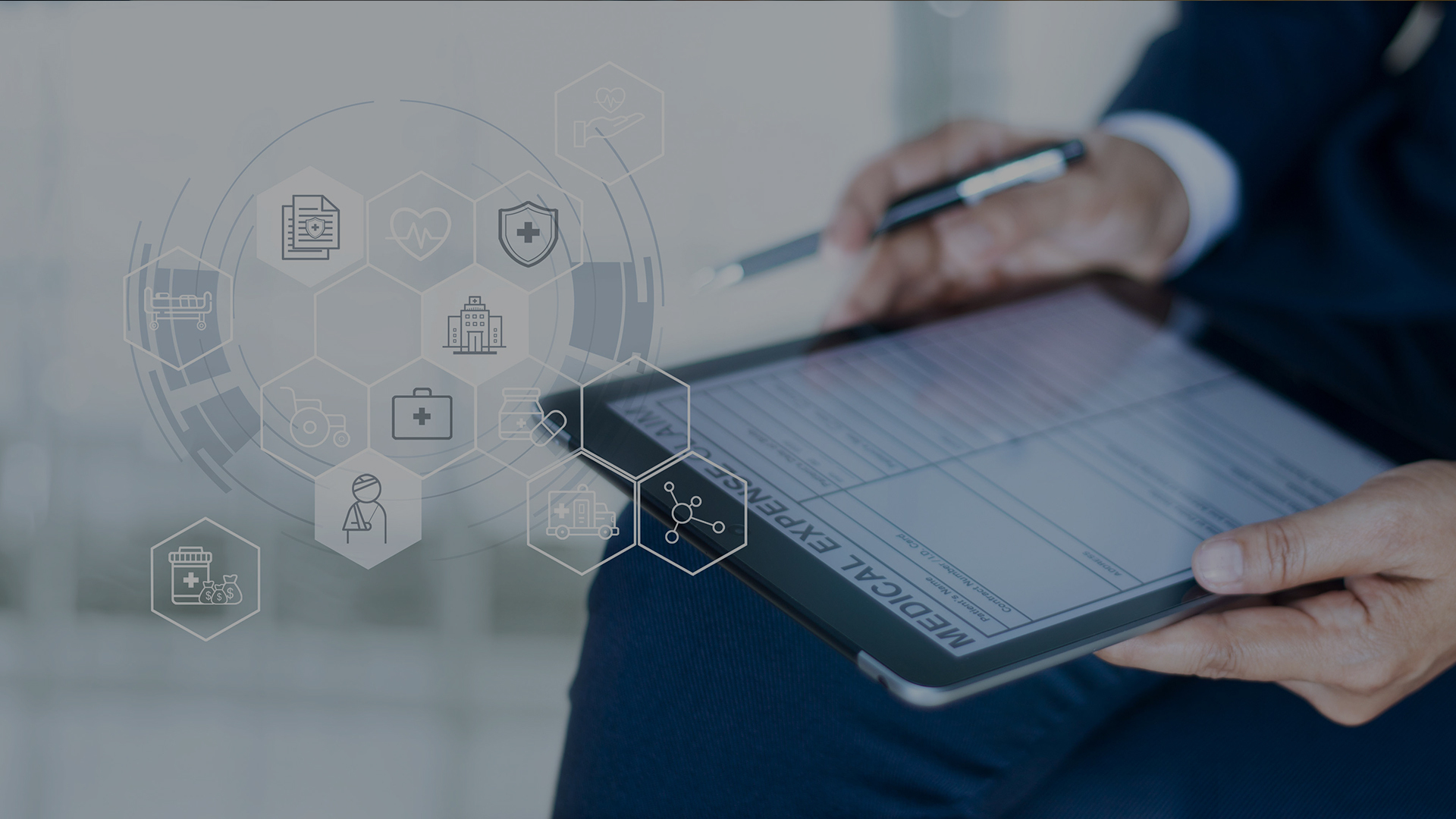
Intelligent Document Processing Automates Data Management for Healthcare Insurer
Claims processing, including back-end services such as adjudication, decisioning, and reimbursement, requires the ingestion and processing of a diverse set of media and complex data types. These can include paper or digital media, images, video, text, and structured and unstructured information. Additionally, each claim form type may have its own associated processing rules.
The customer’s existing approach involved using standard optical character recognition (OCR) with rules-based processing, and led to both large and unwieldy rulesets and a very lengthy exceptions queue. In order to handle the data and deal with the growing list of exception cases, the company relied on manual intervention done by human knowledge workers to make up for their system’s shortcomings.
Manual claims processing is often inefficient and prone to costly and time-consuming errors, especially when complex data is being processed. Reliance on this kind of processing can also stymie growth and make upscaling in times of increased demand more difficult and expensive.
Rule14 implemented a scalable neural network-based document classification engine that dramatically improved the accuracy and speed of document intake and processing. The platform also enabled more generalized field extraction and validation modules in order to enable better utilization of unstructured data in future workflows.
The system optimizes processing costs by providing users with app-level scaling controls and cloud resource utilization insights. It also provides real-time workflow transparency, giving the operations managers greater insight into and control over the active queue, while also helping to identify bottlenecks and other irregularities.
Rule14’s IDP solution was deployed in only three weeks, providing the customer with an unprecedented level of automation via AI-enhanced workflows. For comparison purpose, traditional development technologies and processes would require 6-8 months of development and deployment time.
As part of the solution development, the AI-enhancement team reviewed the end-to-end legacy process and identified key areas where machine learning and advanced models could augment the traditional processing methods to reduce manual effort and increase accuracy and throughput.
The team extracted sample data (averaging around 60,000 images per use case) to train a deep learning-based neural network classifier. The model was tuned to meet or exceed human-level accuracy before production deployment. Accuracy levels are continuously reviewed via statistical process control and audits.
- $10M projected annual savings
- 1 million+ documents processed per month
- 75% automation level achieved, from a baseline of 10% before IDP
- Reduced workflow deployment timeline (2-4 weeks vs. 6-8 months)
- Greater flexibility and scalability - reusable component modules and machine learning models can be rapidly redeployed into new workflows
Discover What Exela's Rule14 Can Do For You